Our Science
Cardiogram — Clinically Validated
DeepHeart: Semi-Supervised Deep Learning for Cardiovascular Risk Prediction
A major challenge for AI in medicine is that labeled training data is costly, scarce, and closely-guarded. DeepHeart is a semi-supervised deep neural network that accurately predicts cardiovascular risk, but requires 10x less labeled data than conventional deep learning techniques.
DeepHeart: Semi-Supervised Sequence Learning for Cardiovascular Risk Prediction. AAAI Conference on Artificial Intelligence, Feb 2018 (AAAI-18)
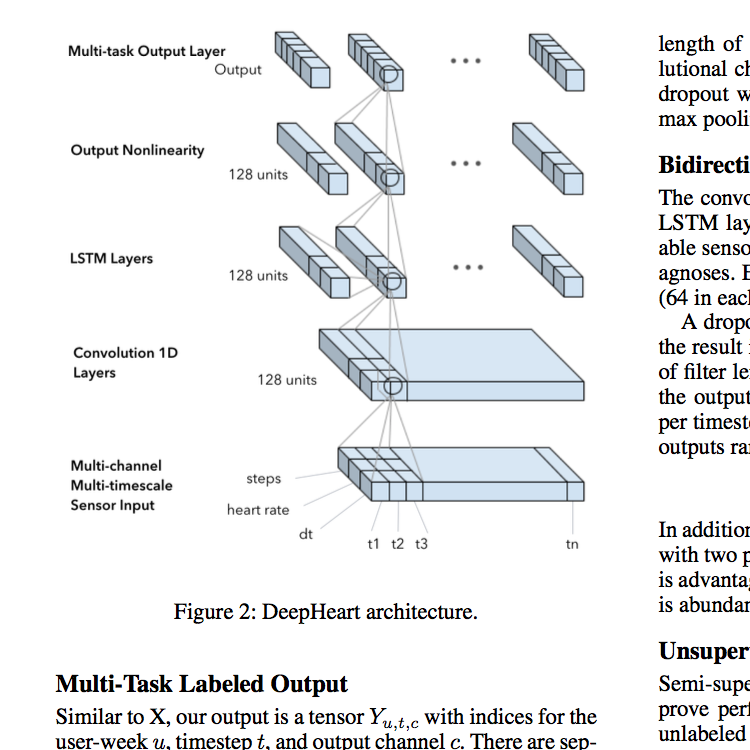
Screening for Atrial Fibrillation
Atrial Fibrillation, the most common abnormal heart rhythm, causes 1 in 4 strokes and frequently goes undiagnosed. In the mRhythm Study, DeepHeart detected atrial fibrillation with 97% accuracy (c-statistic) using optical heart rate sensors, setting the stage for cost-effective, broadly-deployed AF screening.
Detecting Atrial Fibrillation using a Smart Watch - the mRhythm study Heart Rhythm Society Scientific Sessions, May 2017
Passive Detection of Atrial Fibrillation Using a Commercially Available Smartwatch JAMA Cardiology, March 2018
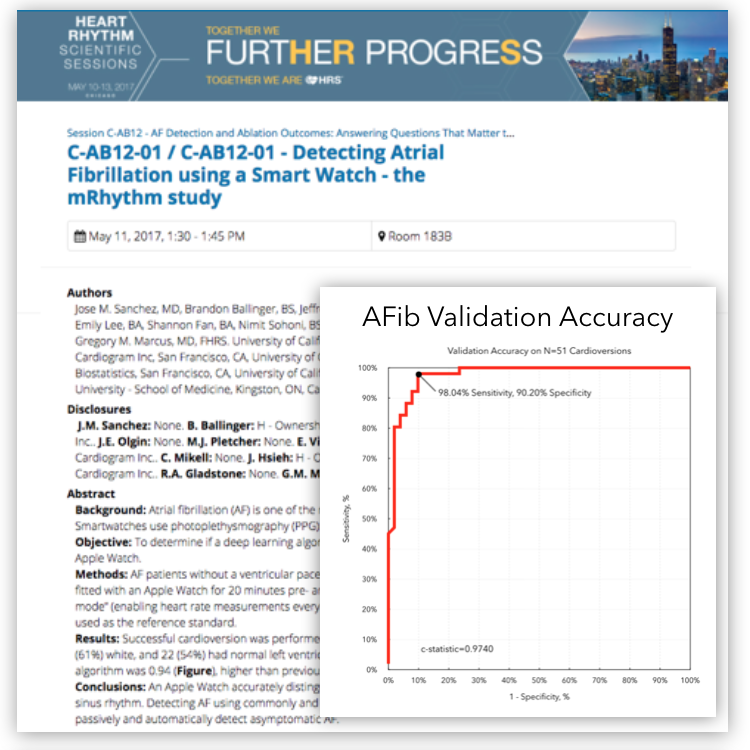
Screening for Sleep Apnea and Hypertension
More than one billion people worldwide have hypertension and sleep apnea. In this study, DeepHeart detected both conditions with more than 82% accuracy using consumer wearables alone.
Cardiovascular Risk Stratification Using Off-the-Shelf Wearables and a Multi-Task Deep Learning Algorithm American Heart Association Scientific Sessions, Nov 2017.
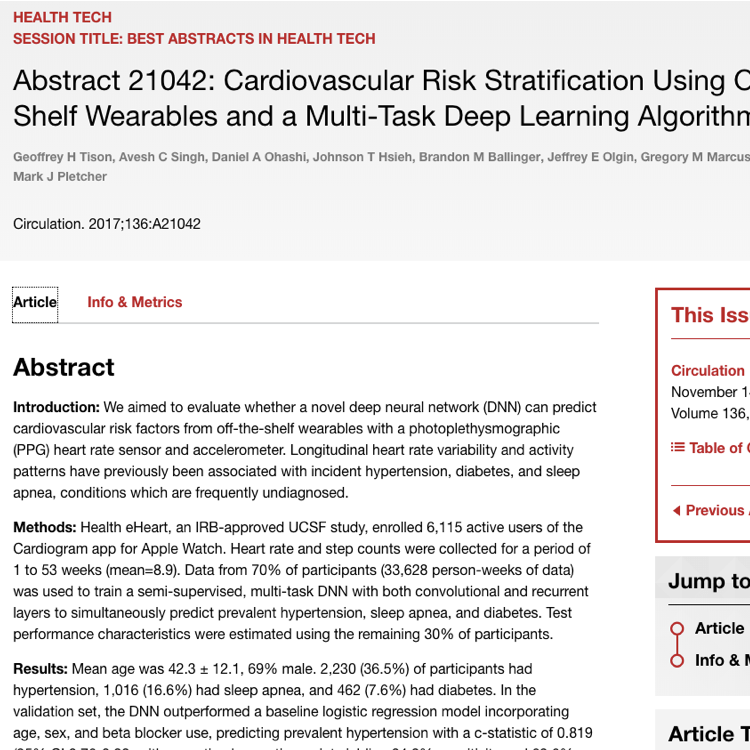
High Engagement Mobile Health Research
The first five ResearchKit apps lost 90% of their users in the first 90 days. By taking inspiration from popular consumer apps, we show it’s possible to improve retention by 5x, exceeding even Twitter and Instagram, with high retention across age groups.
Achieving High Retention in Mobile Health Research Using Design Principles Adopted From Widely Popular Consumer Mobile Apps. American Heart Association Scientific Sessions, Nov 2017
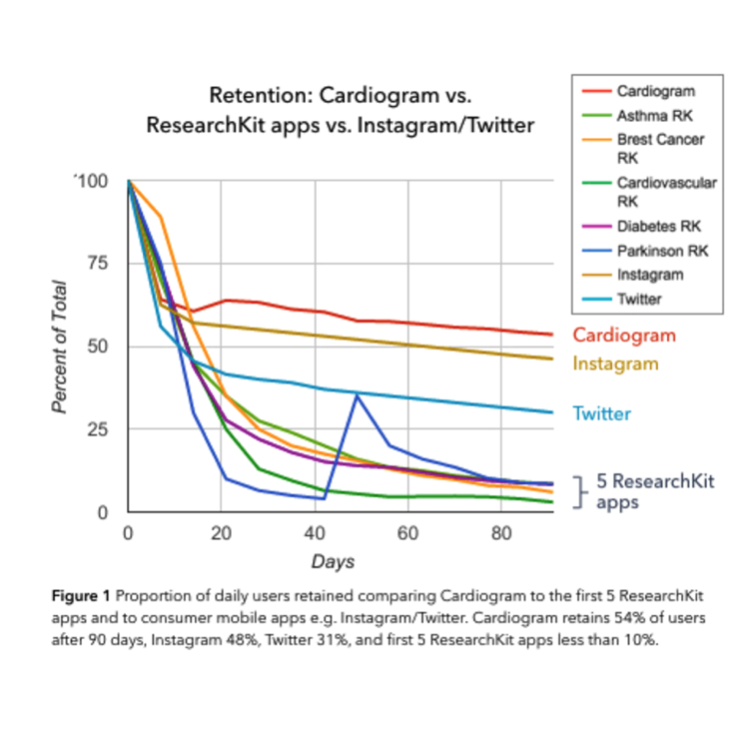