When we talk about artificial intelligence in medicine, we often debate whether machines will replace tasks doctors do today. A more tantalizing possibility is performing tasks doctors can’t—using large data sets, and modern computational tools like deep learning, to recognize patterns too subtle for any human to discern.
Today, we’re presenting early clinical results showing Cardiogram’s deep neural network, DeepHeart, can do just that: recognize hypertension and sleep apnea from wearable heart rate sensors with 82% and 90% accuracy, respectively [1]. The American Heart Association is highlighting this work, conducted in partnership with the UC San Francisco’s Health eHeart Study, as one of three Best Abstracts in Health Tech at their annual AHA Scientific Sessions, a meeting of roughly 18,000 cardiologists.
Globally, 1.1 billion people have hypertension (chronic high blood pressure) and 1 in 5 are undiagnosed. Roughly 24% of men and 9% of women have sleep-disordered breathing, and 80% of people with diagnosable sleep apnea don’t realize they have it. Within the US alone, hypertension and sleep apnea drive $46B and $150B, respectively, in direct medical spend, lost productivity, and accidents.
What if we could transform wearables people already own—Apple Watches, Android Wears, Garmins, and Fitbits—into inexpensive, everyday screening tools using artificial intelligence? That’s what we sought to validate with this new study, alongside N=6,115 participants.
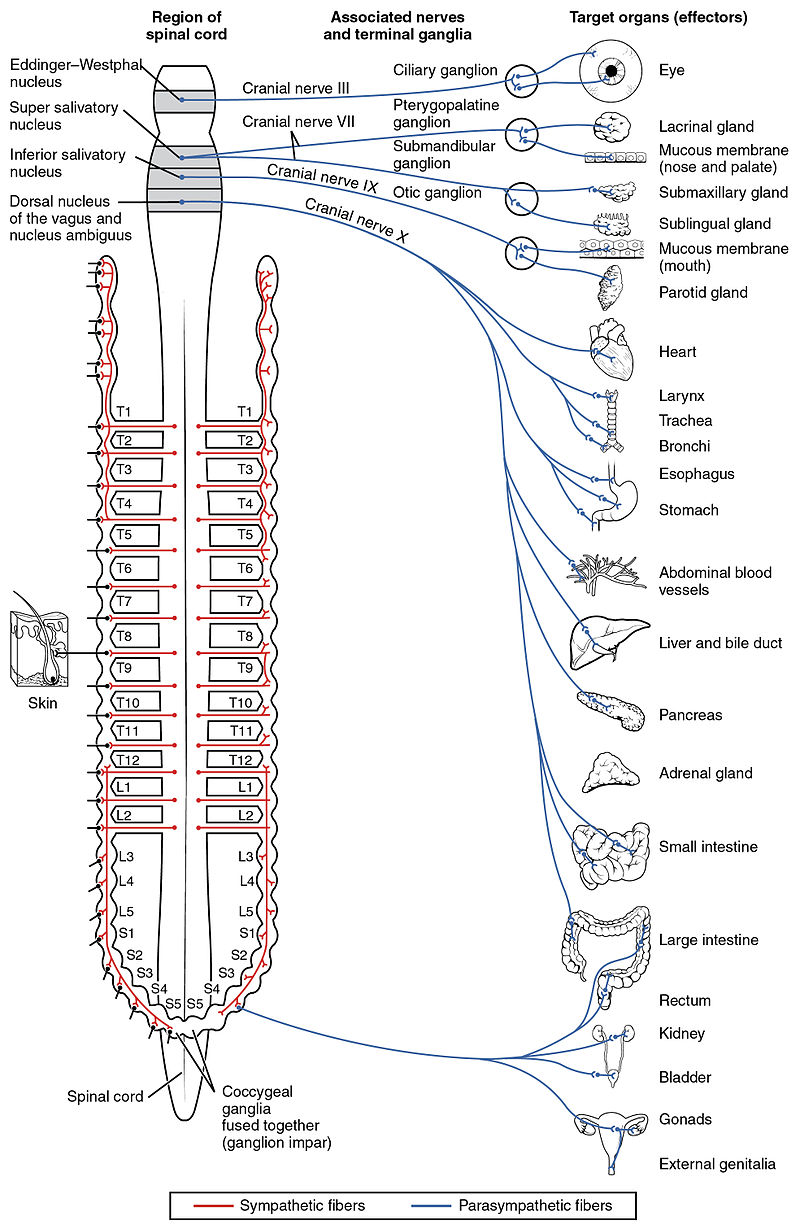
Wait, what? What does heart rate have to do with high blood pressure?
At first glance, the association between heart rate, high blood pressure, and sleep apnea may seem surprising. The connection comes via your autonomic nervous system, which links your heart with your brain, stomach, esophagus, liver, intestines, pancreas, and, importantly, your blood vessels (Fig 1). In 2003, the ARIC study (N=11,061) showed that those with low heart rate variability were 1.44x more likely to develop hypertension over 9 years. The PhysioNet 2000 challenge showed that algorithms based on beat-to-beat heart rate variability could correctly classify 35 recordings of sleep apnea.
These results, based on simple heuristics, were promising, but not applicable without widespread access to heart rate sensors. Within the past two years, major wearable manufacturers like Fitbit, Apple, Garmin, Samsung, Xiaomi, and Android Wear makers have started shipping devices with optical heart rate sensors en masse. Meanwhile, modern AI techniques, such as semi-supervised sequence learning, have become increasingly powerful at extracting hidden patterns from sequential data, like time series of heart rate measurements. Finally, the shift to value-based care means startups can take responsibility for risk end-to-end, removing a major barrier to novel screening methods—fear of high costs due to false positives.
These factors together—new data, new algorithms, and new business models—create an opening to broadly implement preventive medicine based on wearables.
A Novel, Engaged Cardiovascular Cohort
The first challenge in building AI for medicine is getting enough data. Since no existing data set combined wearable data with medical diagnoses, we built our own, recruiting 30,000 participants (and counting) into an online study with the UCSF Health eHeart Study. At the time this study was submitted, 6,115 participants had enrolled, with an average age of 42, 37% having hypertension, and 17% having sleep apnea. As a point of comparison, the original 1948 Framingham Heart Study, which discovered the role of cigarette smoking, blood pressure, and cholesterol in heart disease, followed a total of 5,209 participants.
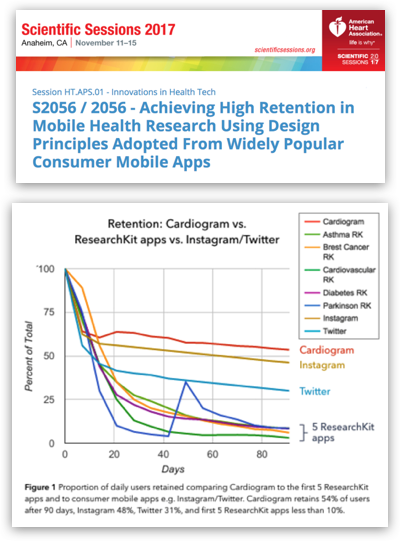
Multi-Task, Semi-Supervised Deep Learning
With data in hand, we could train an algorithm. In May, we presented a study showing DeepHeart could detect atrial fibrillation, the most common abnormal heart rhythm, with 97% accuracy (c-statistic). For this new study, we extended DeepHeart to a multi-task setting — simultaneously predicting the risk of hypertension, sleep apnea, and atrial fibrillation.
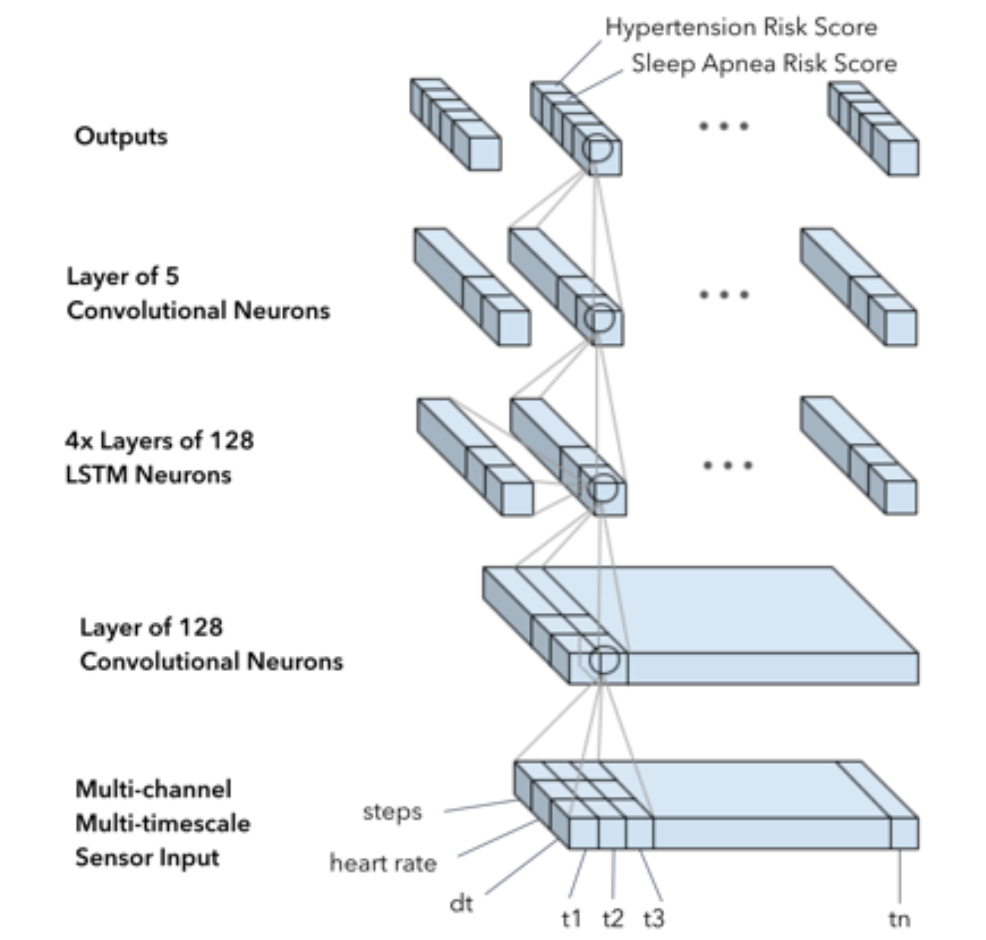
The result was accuracy high enough to support feasible, cost-effective, widely-deployable screening of hypertension and sleep apnea.
For sleep apnea, DeepHeart achieved an accuracy (c-statistic, or AUC ROC) of 90%, with several attractive operating points. For example, we can detect 52% of sleep apnea ( compared to 20% today) with a specificity of 97%. If a specificity of 82% is acceptable, then we can detect even more sleep apnea, about 75% of people. For hypertension, the AUC was 0.82, with an example operating point of 81% sensitivity at 63.2% specificity.
What's next?
Peer-reviewed clinical research is the first step to validate that screening for major health conditions is possible with consumer wearables. But research isn’t the end goal—the end goal is to help real people, in the real world, get diagnosed and treated so that they can live healthier lives.
Over the next few months, you’ll see us start translating these research results into actual care, as well as expand to new conditions like pre-diabetes and diabetes. Stay tuned for future Cardiogram features which will help guide you through clinically-appropriate pathways of diagnosis, treatment, and improved health.